With all the discussions around ChatGPT and Automatic Speech Recognition (ASR) it would seem that Large Language Models (LLMs) as part of a collection of Artificial Intelligence (AI) models could provide us with language understanding capability. Some models appear to be able to answer complex questions based on the amazing amounts of data or information collected from us all. We can speak or type a question into Skype and Bing using ChatGPT and the system will provide us with an uncannily appropriate answer. If we want to speed read through an article the process of finding key words that are meant to represent a main point can also be automated as can a summarisation of content as described in the ‘ChatGPT for teachers: summarize a YouTube transcript’
But can automated processes pick out the words that might help us to remember important points in a transcript, as they are designed to do when manually chosen[1]?
We rarely know where the ASR data comes from and in the case of transcribed academic lectures, the models used tend to be made up of large generic datasets, rather than customised educational based data collections. So, what if the original information the model is gathering is incorrect and the transcript has errors or what if we do not really know what we are looking for when it comes to the main points or we develop our own set of criteria and the automatic key word process cannot support these ideas.
Perhaps it is safe to say that keywords tend to be important elements within paragraphs of text or conversations that could give us clues as to the main theme of an article. In an automatic process they may be missed where there is a two-word synonym such as a ‘bride-to-be’ or ‘future wife’ rather than ‘fiancée’ or a paraphrase or summary that can change the meaning:
Paraphrase: A giraffe can eat up to 75 pounds of Acacia leaves and hay every day.[2]
Original: Giraffes like Acacia leaves and hay and they can consume 75 pounds of food a day.
Keywords are usually names, locations, facts and figures and these can be pronounced in many different ways when spoken by a variety of English speakers[3]. If the system is using a process of randomly learning from its own large language model there maybe few variations in the accents and dialects and perhaps no account of aging voices or cultural settings. These biases have the potential to add yet more errors, which in turn affect the relevance of chosen key words generated through probability models.
Teasing out how to improve the output from AI generated key words is not easy due to the many variables involved. We have already looked at a series of practical metrics in our previous blog and now we have delved into some of the other technological and human aspects that perhaps could help us to understand why automatic key wording is a challenge. A text version of the mind map below is available.
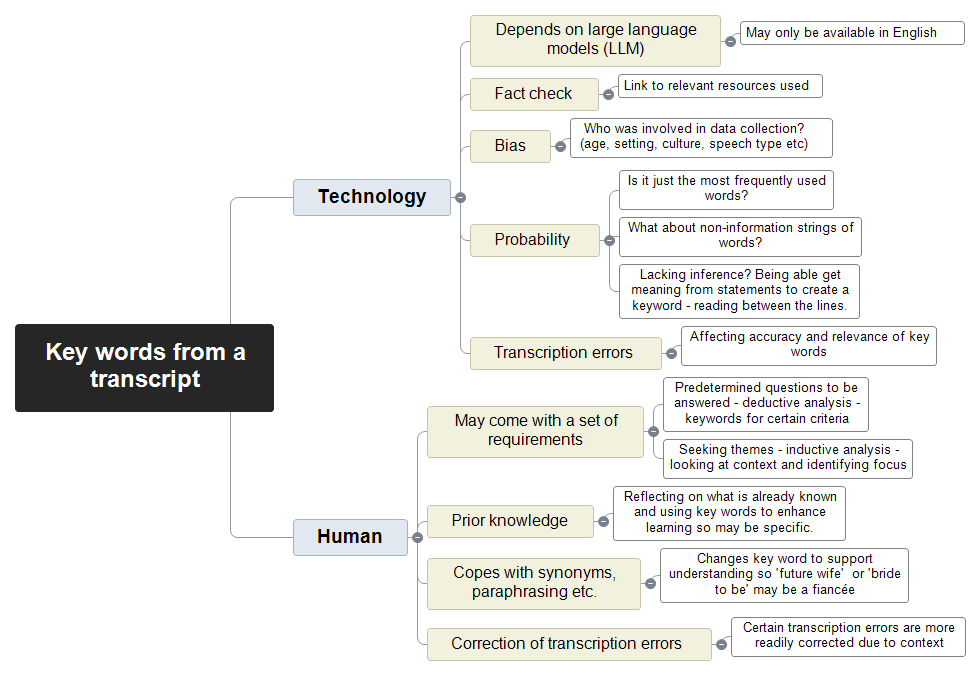
Figure 1. Mind map of key word issues including human aspects, when working with ASR transcripts. Text version available
[1] https://libguides.reading.ac.uk/reading/notemaking
[2] http://www.ocw.upj.ac.id/files/Slide-LSE-04.pdf
[3] https://aclanthology.org/2022.lrec-1.680.pdf