This is just the start of a user needs analysis related to the way we think about Automatic Speech Recognition (ASR) challenges and our requirements for captions and transcripts to help those watching and listening to streaming videos and audio.
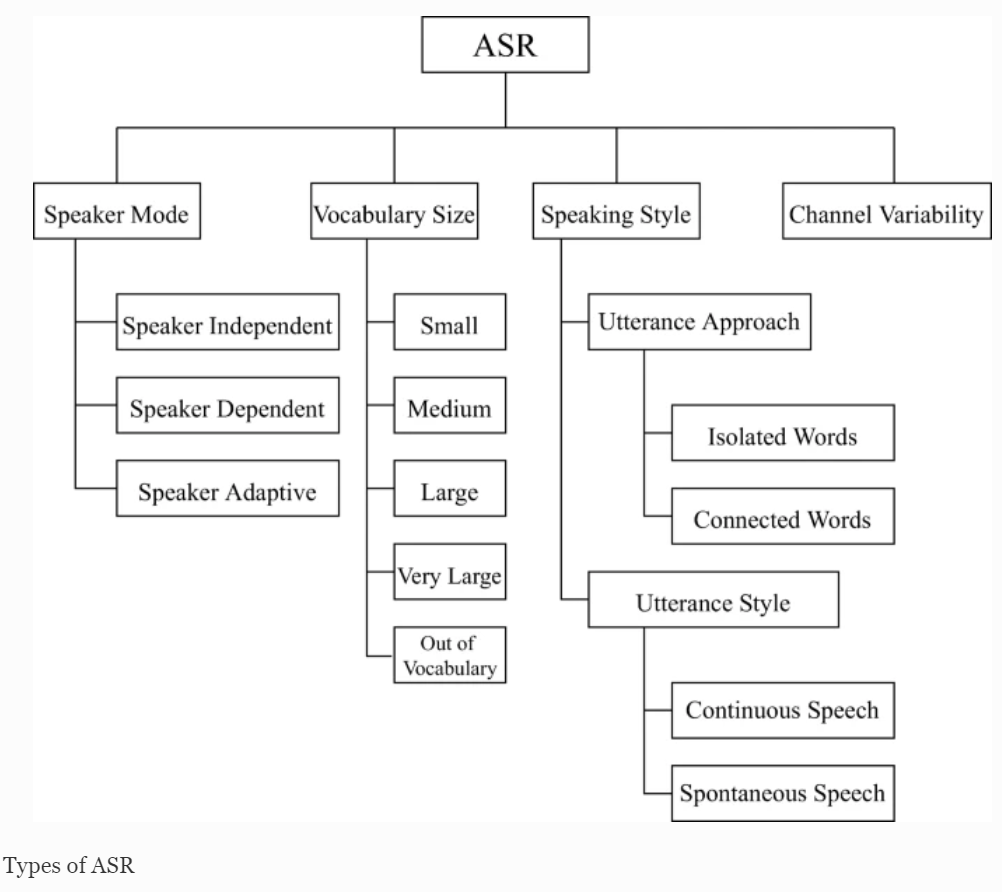
Working from left to right in the above diagram the authors of this paper ask us to think about a ‘Speaker Mode’ such as a trained speech pattern from a sole user who, with a good speaking style, can result in good ASR results. This mode is compared to an untrained speech pattern from one person or multiple speakers with overlapping conversations which can be harder for ASR models. Moving across to the ‘Vocabulary Size’ used in the conversations or presentations, there may be large and complex sections of text which, when considered alongside different ‘Speaking Styles’, can also affect output. ‘Channel Variability’, such as a noisy environment is a further challenge. The technologies used (microphones and cameras) may also be unsuitable for the best results. Finally, latency when live streaming ASR involves the time it takes for data to get to the ASR servers and back again. This process also includes how long the transcribing takes. Both types of latency has an impact on the accuracy of captions and transcriptions.
However, having accepted all these challenges and several other issues that may have been missed, there is the essential task of thinking about user needs. In our case this involves student needs and the desire for increased flexibility in the way learning occurs at a higher education level. There remains the wish to have 100% accurate captions that fit the timeline of a conversation or presentation / lecture, but some users may wish to have different size fonts or colours as the text crosses the screen, others may wish to have key words highlighted. Then there are the transcripts that can offer easier reading by omitting many of the natural non-word insertions such as ‘ums’ and ‘uhs’, but need to accurately capture multiple acronyms to help understanding, so they can be defined at a later date.
As we explore the way applications present captions and transcripts, it is not surprising that there seem to very few discussions about personalisation and customisation of captions and transcripts for educational use. Most students are provided with instructions for the various systems, with little choice as to whether they wish to have slides on show or hidden, titles in larger text and paragraphs with timings. Then it may be important for all the inserted non-words to be left in place because they can add to the emotional content of a presentation. Some captions are offered as a stream of text as a type of transcript. This is how they are provided by YouTube, including all the timings down to two seconds apart, with phrases in long columns. The content can be copied and pasted into a document, but it is not that easy to read for note taking, with very minimal punctuation and liberal capturing of non-word insertions.
Other systems such as Otter.ai offer a downloadable transcript that has speaker recognition and key points as an outline targeted for use in meetings. These options could be handy for lecture notes. The system strips out any non-word insertions, adds punctuation and copes well with many complex words and different speakers, giving each one a number.
As a way of personalising video viewing and lecture capture, it is possible to combine the YouTube experience with captions by then converting the MP4 video file (if it is downloadable) into an MP3 format (free VLC offers this option) or use a web service and then upload it to Otter.ai as a free trial! It really does not take long and the process allows me to analyse Word Error Rates for testing accuracy, by comparing documents as well as using highlighting and adding annotations!
Warning: Obviously because the process described involves AI and automation, not every aspect of the captions or transcript output can be guaranteed to be 100% correct, so there is still the need to have manual checks in place if a verbatim output is required.